Qualitative Data Analysis
21 Qualitative Coding
Mikaila Mariel Lemonik Arthur
Codes are words or phrases that capture a central or notable attribute of a particular segment of text or visual data (Saldaña 2016). Coding, then, is the process of applying codes to texts or visuals. It is one of the most common strategies for data reduction and analysis of qualitative data, though many qualitative projects do not require or use coding. This chapter will provide an overview of approaches based in coding, including how to develop codes and how to go through the coding process.
In order to understand coding, it is essential to think about what it means for something to be a code. To analogize to social media, codes might function a bit like tags or hashtags. They are words or phrases that convey content, ideas, perspectives, or other key elements of segments of text. Codes are not the same as themes. Themes are broader than codes—they are concepts or topics around which a discussion, analysis, or text focuses. Themes are more general and more explanatory—often, once we code, we find themes emerge as ideas to explore in our further analysis (Saldaña 2016). Codes are also different from descriptors. Descriptors are words or phrases that describe characteristics of the entire text and/or the person who created it. For example, if we note the profession of an interview respondent, whether an article is news or opinion, or the type of camera used to take a photograph, those would be descriptors. Saldaña (2016) instead calls these attributes. The term attributes more typically refers to the possible answer choices or options for a variable, so it is possible to think about descriptors as variables (or perhaps their attributes) as well.
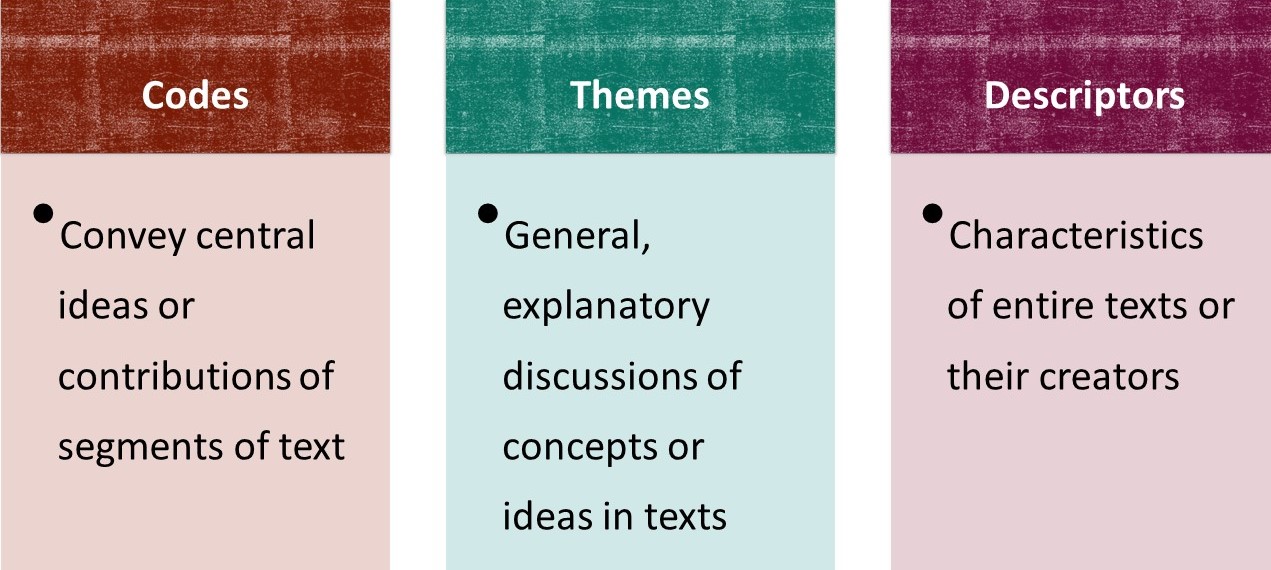
Let’s consider an example. Imagine that you were conducting an interview-based study looking at minor-league athletes’ workplace experiences and later-life career plans. In this study, themes might be broad ideas like “aspirations” or “work experiences.” There would be a vast array of codes, but they might include things like “short-term goals,” “educational plans,” “pay,” “team bonding,” “travel,” “treatment by managers,” “family demands,” and many more. Descriptors might include the athlete’s gender and what sport they play.
Developing a Coding System
While all approaches to coding have in common the idea that codes are applied to segments of text or visuals, there are many different ways to go about coding. These approaches differ in terms of when they occur during the research process and how codes are developed. First of all, there is a distinction between first- and second-cycle coding approaches (Saldaña 2016). First-cycle coding happens early in the research process and is really a bridge from data reduction to data analysis, while second-cycle coding occurs later in the research process and is more analytical in nature. Another version of this distinction is the comparison between rough, analytic, and focused coding. Rough coding is really part of the process of data reduction. It often involves little more than putting a few words near each segment of text to make clear what is important in that segment, with the approach being further refined as coding continues. In contrast, analytic coding involves more detailed techniques designed to move towards the development of themes and findings. Finally, focused coding involves selecting ideas of interest and going back and re-coding your texts to orient your approach more specifically around these ideas (Bergin 2018).
A second set of distinctions concerns whether the data drives the development of codes or whether codes are instead developed in advance. If codes are determined in advance, or predetermined, researchers develop a set of codes based on their theory, hypothesis, or research question. This sort of coding is typically called deductive coding or closed coding. In contrast, open coding or inductive coding refers to a process in which researchers develop codes based on what they observe in their data, grounding their codes in the texts. This second approach is more common, though by no means universal, in qualitative data analysis. In both types of coding, however, researcher may rely upon ideas generated by writing theoretical memos as they work through the connections between concepts, theory, and data (Saldaña 2016).
Finally, a third set of distinctions focuses on what is coded. Manifest coding refers to the coding of surface-level and easily observable elements of texts (Berg 2009). In contrast, latent coding is a more interpretive approach based on looking deeply into texts for the meanings that are encoded within or symbolized by them (Berg 2009). For example, consider a research project focused on gender in car advertisements. A manifest approach might count the number of men versus women who appear in the ads. A latent approach would instead focus on the use of gendered language and the extent to which men and women are depicted in gender-stereotyped ways.
Researchers need to answer two more questions as they develop their coding systems. First, what to code, and second, how many codes. When thinking about what to code, researchers can look at the level of individual words, characters or actors in the text, paragraphs, entire textual items (like complete books or articles), or really any unit of text (Berg 2009), but the most useful procedure is to look for chunks of words that together express a thought or idea, here referred to as “segments of text” or “textual segments,” and then code to represent the ideas, concepts, emotions, or other relevant thoughts expressed in those chunks.
How many codes should a particular coding system have? There is no simple answer to this question. Some researchers develop complex coding systems with many codes and may have over a hundred different codes. Others may use no more than 25, perhaps fewer, even for the same size project (Saldaña 2016). Some researchers nest codes into code trees, with several related “child” codes (or subcodes) under a single “parent” code. For example, a code “negative emotions” could be the parent code for a series of codes like “anger,” “frustration,” “sadness,” and “fear.” This approach enables researcher to use a smaller or larger number of codes in their analysis as seems fit after coding is complete. While there is no formula for determining the right number of codes for a particular project, researchers should be attentive to overgrowth in the number of codes. Codes have limited analytical value if they are used only once or twice—if a coding system includes many codes that are applied only a small number of times, consider whether there are larger categories of codes that might be more useful. Occasionally, there are codes worth keeping but applying rarely, for example when there is a rare but important phenomenon that arises in the data. But for the most part, codes should be used with some degree of frequency in order for them to be useful for uncovering themes and patterns.
Types of Codes
A wide variety of different types of codes can be used in coding systems. The discussion below, which draws heavily on the work of Saldaña (2016), details a variety of different approaches to coding and code development. Researchers do not need to choose just one of these approaches—most researchers combine multiple coding approaches to create an overall system that is right for the texts they are coding and the project they are conducting. The approaches detailed here are presented roughly in order of the degree of complexity they represent.
At the most basic level is descriptive coding. Descriptive codes are nouns or phrases describing the content covered in a segment of text or the topic the segment of text focuses on. All studies can use descriptive coding, but it often is less productive of rich data for analysis than other approaches might be. Descriptive coding is often used as part of rough coding and data reduction to prepare for later iterations of coding that delve more deeply into the texts. So, for instance, that study of sexism in advertisements might involve some rough coding in which the researcher notes what type of product or service is being advertised in each advertisement.
Structural coding, in contrast, attends more closely to the research question rather than to the ideas in the text. In structural coding, codes indicate which specific research question, part of a research question, or hypothesis is being addressed by a particular segment of text. This may be most useful as part of rough coding to help researchers ensure that their data addresses the questions and foci central to their project.
In vivo coding captures short phrases derived from participants’ own language, typically action-oriented. This is particularly important when researchers are studying subcultural groups that use language in different ways than researchers are accustomed to and where this language is important for subsequent analysis (Manning 2017). In this approach, researchers choose actual portions of respondents’ words and use those as codes. In vivo coding can be used as part of both rough and analytical coding processes.
A related approach is process coding, which involves “the use of gerunds to label actual or conceptual actions relayed by participants” (Saldaña 2016:77). (Gerunds are verb forms that end in -ing and can function grammatically as if they are nouns when used in sentences). Process coding draws researchers’ attention to actions, but in contrast to in vivo coding it uses the researcher’s vocabulary to build the coding system. So, for instance, in the study of minor league athletes discussed earlier in the chapter, process codes might include “traveling,” “planning,” “exercising,” “competing,” and “socializing.”
Concept coding involves codes consisting of words or short phrases that represent broader concepts or ideas rather than tangible objects or actions. Sticking with the minor league athletes example, concept codes might include “for the love of the game,” “youth,” and “exploitation.” A combination of concept, process, and descriptive coding may be useful if researchers want their coding system to result in an inventory of the ideas, objects, and actions discussed in the texts.
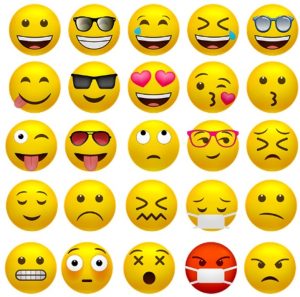
Emotion codes are codes indicating the emotions participants discuss in or that are evoked by a segment of text. A more contemporary version of emotion codes relies on “emoticodes” or the emoji that express specific kinds of emotions, as shown in Figure 2.
Values coding involves the use of codes designed to represent the “perspectives or worldview” of a respondent by conveying participants’ “values, attitudes, and beliefs” (Saldaña 2016:131). For example, a project on elementary school teachers’ workplace satisfaction might include values codes like “equity,” “learning,” “commitment,” and “the pursuit of excellence.” Do note that choices made in values coding are, even more so than in other forms of coding, likely to reflect the values and worldviews of the coder. Thus, it can be essential to use a team of multiple coders with different backgrounds and perspectives in order to ensure a values coding approach that reflects the contents of the texts rather than the ideas of the coders.
Versus coding requires the construction of a series of binary oppositions and then the application of one or the other of the items in the binary as a code to each relevant segment of text. This may be a particularly useful approach for deductive coding, as the researcher can set out a series of hypothesized binaries to use as the basis for coding. For example, the project on elementary school teachers’ workplace satisfaction might use binaries like feeling supported vs. feeling unsupported, energized vs. tired, unfulfilled needs vs. fulfilled needs, kids ready to learn vs. kids needing services, academic vs non-academic concerns, and so on.
Evaluation coding is used to signify what is and is not working in the policy, program, or endeavor that respondents are discussing or that the research focuses on. This approach is obviously especially useful in evaluation research designed to assess the merit or functioning of particular policies or programs. For example, if the project about elementary school teachers was part of a mentoring program designed to keep new teachers in the education profession, codes might include “future orientation” to flag portions of the text in which teachers discuss their longer-term plans and “mentor/mentee match” to flag portions in which they explore how they feel about their mentors, both key elements of the program and its goals.
There are a variety of other approaches more common outside of sociology, such as dramaturgical coding, which is a coding approach that treats interview transcripts or fieldnotes as if they are scripts for a play, coding such things as actors, attitudes, conflicts, and subtexts; coding approaches relying on terms and ideas from literary analysis; and those drawn from communications studies, which focus on facets of verbal exchange. Finally, some researchers have outlined very specific coding strategies and procedures such that someone else could pick up their methods and apply them exactly. This sort of approach is typically deductive, as it requires the advance specification of the decisions that will be made about coding.
Some coding strategies incorporate measures of weight or intensity, and this can be combined with many of the approaches detailed above. For example, consider a project collecting narratives of people’s experiences with losing their jobs. Respondents might include a variety of emotional content in their narratives, whether sadness, fear, stress, relief, or something else. But the emotions they discuss will vary not only in type, they will also vary in extent. A worker who is fired from a job they liked well enough but who knows they will be able to find another job soon may express sadness while a worker whose company closed after she worked there for 20 years and who has few other equivalent employment opportunities in the region may express devastation. Code weights help account for these differences.
A final question researchers must consider is whether they will apply only one code per segment of text or will permit overlapping codes. Overlapping codes make data analysis more complex but can facilitate the process of looking for relationships between different concepts or ideas in the data.
Codebooks
As a coding system is developed and certainly upon its completion, researchers create documents known as codebooks. As is the case with survey research, codebooks lay out the details of how the measurement instrument works to capture data and measure it. For surveys, a codebook tells researchers how to transform the multiple-choice and short-answer responses to survey questions into the numerical data used for quantitative analysis. For qualitative coding, codebooks instead explain when and how to use each of the codes included in the project. Codebooks are an important part of the coding process because they remind the researcher, and any other coders working on the project, what each code means, what types of data it is meant to apply to, and when it should and should not be used (Luker 2008). Even if a researcher is coding without others, it is easy to lose sight of what you were thinking when you initially developed your coding system, and so the codebook serves as an important reminder.
For each code, the codebook should state the name of the code, include a couple of sentences describing the code and what it should be used for, any information about when the code should not be used, examples of both typical and atypical conditions under which the code would be used, and a discussion of the role the code plays in analysis (Saldaña 2016). Codebooks thus serve as instruction manuals for when and how to apply codes. They can also help researchers think about taxonomies of codes as they organize the code book, with higher-level ideas serving as categories for groups of child, or more precise, codes.
The Process of Coding
So, what does the process of coding look like? While qualitative research can and does involve deductive approaches, the process that will be detailed here is an inductive approach, as this is more common in qualitative research. This discussion will lay out a series of steps in the coding process as well as some additional questions researchers and analysts must consider as they develop and carry out their coding.
The first step in inductive coding is to completely and thoroughly read through the data several times while taking detailed notes. To Saldaña (2016), the most important question to ask during this initial read is what is especially interesting or surprising or otherwise stands out. In addition, researchers might contemplate the actions people take, how people go about accomplishing things, how people use language or understand the world, and what people seem to be thinking. The notes should include anything and everything—objects, people, emotions, actions, theoretical ideas, questions—really anything, whether it comes up again and again in the data or only once, though it is useful to flag or highlight those concepts that seem to recur frequently in the data.
Next, researchers need to organize these notes into a coding system. This involves deciding which coding approach(es) to incorporate, whether or not to use parent and child codes, and what sort of vocabulary to use for codes. Remember that readers will not see the coding system except insofar as the researcher chooses to convey it, so vocabulary and terms should be chosen based on the extent to which they make sense to the research team. Once a coding system has been developed, the researcher must create a codebook. If paper coding will be used, a paper codebook should be created. If researchers will be using CAQDAS, or computer-aided qualitative data analysis software, to do their coding, it is often the case that the codebook can be built into the software itself.
Next, the researcher or research team should rough code, applying codes to the text while taking notes to reflect upon missing pieces in the coding system, ways to reorganize the codes or combine them to enhance meaning, and relevant theoretical ideas and insights. Upon completing the rough coding process, researchers should revise the coding system and codebook to fully reflect the data and the project’s needs.
At this point, researchers are ready to engage in coding using the revised codebook. They should always have someone else code a portion of the texts—usually a minimum of 10%—for interrater reliability checks, and if a larger research team is used, 10% of the texts should be coded in common by all coders who are part of the research team. Even in cases where researchers are working alone, it truly strengthens data analysis to be able to check for interrater reliability, so most analysts suggest having a portion of the data coded by another coder, using the codebook. If at all possible, additional coding staff should not be told what the hypothesis or research question is, as one of the strengths of this approach is that additional coding staff will be less likely to be influenced by preexisting ideas about what the data should show (Luker 2008). There are various quantitative measures, such as Chronbach’s alpha and Kappa, that researchers use to calculate interrater reliability, the measure of how closely the ratings of multiple coders correspond. All coders should keep detailed notes about their coding process and any obstacles or difficulties they encounter.
How do researchers know they are done coding? Not just because they have gone through each text once or twice! Researchers may need to continue repeating this process of revision and re-coding until additional coding does not reveal anything more. This repetition is an essential part of coding, as coding always requires refinement and rethinking (Saldaña 2016). In Berg’s (2009:354-55) words, it is essential to “code minutely,” beginning with a rough view of the entire text and then refining as you go until you are examining each detail of a text. Then, researchers think about why and how they developed their codes and what jumps out at them as important from the research as they delve into findings, making sure that nothing has been left out of the coding process before they move towards data analysis.
One interesting question is whether the identities and standpoints (as discussed in the chapter “The Qualitative Approach”) of coders matter to the coding process. Eduardo Bonila-Silva (Zuberi and Bonilla-Silva 2008:17) has described how, after a presentation discussing his research on racism, a colleague asked whether the coders were White or Black—and he responded by asking the colleague “if he asked such questions across the board or only to researchers saying race matters.” As Bonilla-Silva’s question suggests, race (like other aspects of identity and experience, such as gender, immigration status, disability status, age, and social class, just to name a few) very well might shape the way coders see and understand data, functioning as part of a particular coding filter (Saldaña 2016). But that shaping extends broadly across all issues, not just those we might assume are particularly salient in relationship to identities. Thus, it is best for research teams to be diverse so as to ensure that a variety of perspectives are brought to bear on the data and that the findings reflect more than just a narrow set of ideas about how the world works.
Coding and What Comes After
If researchers will code by hand, they will need multiple copies of their data, one for reference and one for writing on (Luker 2008). On the copy that will be written on, researchers use a note-taking system that makes sense to them—whether different-colored markers, Roman numerals in the margins, a complex series of sticky notes, or whatever—to mark the application of various codes to sections of your data. You can see an example of what hand coding might look like in Figure 3 below, which is taken from a study of the comments faculty members make on student writing. Segments of text are highlighted in different colors, with codes noted in the margins next to the text. You can see how codes are repeated but in different combinations. Once the initial coding process is complete, researchers often cut apart the pieces of paper to make chunks of text with individual codes and sort the pieces of paper by code (if multiple codes appear in individual chunks of text, additional copies might be needed). Then, each pile is organized and used as the basis for writing theoretical memos. Another option for coding by hand is to use an index sheet (Berg 2009). This approach entails developing a set of codes and categories, arranging them on paper, and entering transcript, page, and paragraph information to identify where relevant quotes can be found.
For more complex analytical processes, researchers will likely want to use software, though there are limitations to software. Luker (2008), for instance, argues that when coding manually, she tends to start with big themes and only breaks them into their constituent parts later, while coding using software leads her to start with the smallest possible codes. (One solution to this, offered by some software packages, is upcoding, where a so-called “parent” code is simultaneously applied to all of the “child” codes under it. For instance, you might have a parent code of “activism” and then child codes that you apply to different kinds of activism, whether protest, legislative advocacy, community organizing, or whatever.)
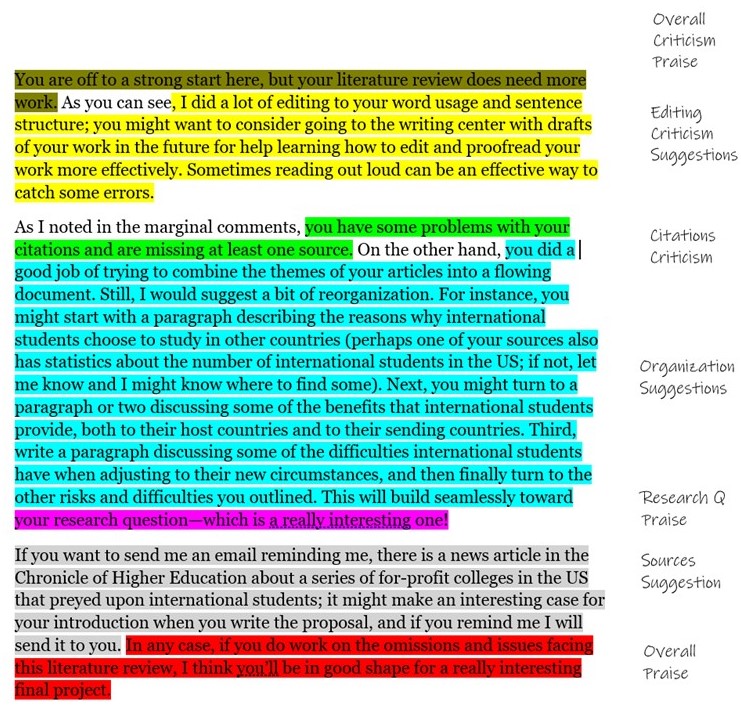
Coding does not stand on its own, and thus simply completing the coding process does not move a research project from data to analysis. While the analysis process will be discussed in more detail in a subsequent chapter, there are several steps researchers take alongside coding or immediately after completing coding that facilitate analysis and are thus useful to discuss in the context of coding. Many of these are best understood as part of the process of data reduction. One of the most important of these is categorizing codes into larger groupings, a step that helps to enable the development of themes. These larger groupings, sometimes called “parent” codes, can collapse related but not identical ideas. This is always useful, but it is especially useful in cases where researchers have used a large number of codes and each one is applied only a few times. Once parent codes have been created, researchers then go back and ensure that the appropriate parent code is assigned to all segments of text that were initially coded with the relevant “child” codes (a step that can be automated in CAQDAS). If appropriate, researchers may repeat this process to see if parent codes can be further grouped. An alternative approach to this grouping process is to wait until coding is complete, and then create more analytical categories that make sense as thematic groupings for the codes that have been utilized in the project so far (Saldaña 2016).
There are a variety of other approaches researchers may take as part of data reduction or preliminary analysis after completing coding. They may outline the codes that have occurred most frequently for specific participants or texts, or for the entire body of data, or the codes that are most likely to co-occur in the same segment of text or in the same document. They may print out or photocopy documents or segments of text and rearrange them on a surface until the arrangement is analytically meaningful. They may develop diagrams or models of the relationships between codes. In doing this, it is especially helpful to focus on the use of verbs or other action words to specify the nature of these relationships—not just stating that relationships exist, but exploring what the relationships do and how they work.
In inductive coding especially, it is often useful to write theoretical and analytical memos while coding occurs, and after coding is completed it is a good time to go back and review and refine these memos. Here, researchers both clearly articulate to themselves how the coding process occurred and what methodological choices they made as well as what preliminary ideas they have about analysis and potential findings. It can be very useful to summarize one’s thinking and any patterns that might have been observed so far as a step in moving towards analysis. However, it is extremely important to remember the data and not just the codes. Qualitative researchers always go back to the actual text and not just the summaries or categories. So a final step in the process of moving toward analysis might be to flag quotes or data excerpts that seem particularly noteworthy, meaningful, or analytically useful, as researchers need these examples to make their data come alive during analysis and when they ultimately present their results.
Becoming a Coder
This chapter has provided an overview of how to develop a coding system and apply that system to the task of conducting qualitative coding as part of a research project. Many new researchers find it easy—if sometimes time-consuming and not always fascinating—to get engaged with the coding process. But what does it take to become an effective coder? Saldaña (2016) emphasizes personality attributes and skills that can help. Some of these are attributes and skills that are important for anyone who is involved in any aspect of research and data analysis: organization, to keep track of data, ideas, and procedures; perseverance, to ensure that one keeps going even when the going is tough, as is often the case in research; and ethics, to ensure proper treatment of research participants, appropriate data security behaviors, and integrity in the use of sources. In most aspects of data analysis, creativity is also important, though there are some roles in quantitative data analysis that require more in the way of technical skills and ability to follow directions. In qualitative data analysis, creativity remains important because of the need to think deeply and differently about the data as analysis continues. Flexibility and the ability to deal with ambiguity are much more important in qualitative research, as the data itself is more variable and less concrete; quantitative research tends to place more emphasis on rules and procedures. A final strength that is particularly important for those working in qualitative coding is having a strong vocabulary, as vocabulary both helps researchers understand the data and enhances their ability to create effective and useful coding systems. The best way to develop a stronger vocabulary is to read more, especially within your discipline or field but broadly as well, so researchers should be sure to stay engaged with reading, learning, and growing.
Reading, learning, and growing, along with a lot of practice, is of course how researchers enhance their data collection, coding, and data analysis skills, so keep working at it. Qualitative research can indeed be easy to get started with, but it takes time to become an expert. Put in the time, and you, too, can become a skilled qualitative data analyst.
Exercises
- For each of the following words or phrases, consider whether it is most likely to represent a code, a theme, or a descriptor. Explain your response.
- Female respondent
- Energized
- The relationship between poverty and social control
- Creative
- A teacher
- The process of divorce
- Social hierarchies
- Grief
- Pick a research topic you find interesting and determine which of the approaches to coding detailed in this chapter might be most appropriate for your topic, then write a paragraph about why this approach is the best.
- Sticking with the same topic you used to respond to Exercise 2, brainstorm some codes that might be useful for coding texts related to this topic. Then, write appropriate text for a codebook for each of those codes.
- Select a hashtag of interest on a particular social media site and randomly sample every other post using that hashtag until you have selected 15 tweets. Then inductively code those posts and engage in summarization or classification to determine what the most important themes they express might be.
- Create a codebook based on what you did in Exercise 4. Exchange codebooks and tweets with a classmate and code each other’s tweets according to the instructions in the codebook. Compare your results—how often did your coding decisions agree and how often did they disagree? What does this tell you about interrater reliability, codebook construction, and coder training?
Media Attributions
- codes themes descriptors © Mikaila Mariel Lemonik Arthur is licensed under a CC BY-NC (Attribution NonCommercial) license
- Emoticodes © AnnaliseArt is licensed under a CC BY (Attribution) license
- Hand Coding Example © Mikaila Mariel Lemonik Arthur is licensed under a CC BY-NC-ND (Attribution NonCommercial NoDerivatives) license
Words or phrases that capture a central or notable attribute of a particular segment of textual or visual data.
The process of assigning observations to categories.
Concepts, topics, or ideas around which a discussion, analysis, or text focuses.
A category in an information storage system; more specifically in Dedoose, a characteristic of an author or entire text. Also, the word used to indicate that category or characteristic.
The possible levels or response choices of a given variable.
Coding that occurs early in the research process as part of a bridge from data reduction to data analysis.
Analytical coding that occurs later in the data analysis process.
Coding for data reduction or as part of an initial pass through the data.
Coding designed to move analysis towards the development of themes and findings.
Selective coding designed to orient an analytical approach around certain ideas.
Coding in which the researcher developed a coding system in advance based on their theory, hypothesis, or research question.
Coding in which the researcher developed a coding system in advance based on their theory, hypothesis, or research question.
Coding in which the researcher develops codes based on what they observe in the data they have collected.
Coding in which the researcher develops codes based on what they observe in the data they have collected.
Coding of surface-level and/or easily observable elements of texts.
Interpretive coding that focuses on meanings within texts.
Coding that relies on nouns or phrases describing the content or topic of a segment of text.
Coding that indicates which research question or hypothesis is being addressed by a given segment of text.
Coding that relies on research participants' own language.
Coding in which gerunds are applied to actions that are described in segments of text.
Verb forms that end in -ing and function grammatically in sentences as if they are nouns.
Coding using words or phrases that represent concepts or ideas.
Codes indicating emotions discussed by or present in the text, sometimes indicated by the use of emoji/emoticons.
Coding that relies on codes indicating the perspective, worldview, values, attitudes, and/or beliefs of research participants.
Coding that relies on a series of binary oppositions, one of which must be applied to each segment of text.
A coding system used to indicate what is or is not working in a program or policy.
Coding that treats texts as if they are scripts for a play.
Elements of a coding strategy that help identify the intensity or degree of presence of a code in a text.
Documents that lay out the details of measurement. Codebooks may be used in surveys to indicate the way survey questions and responses are entered into data analysis software. Codebooks may be used in coding to lay out details about how and when to use each code that has been developed.
A measure of association especially likely to be used for testing interrater reliability.